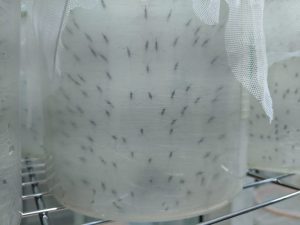
Diseases transmitted by mosquito vectors such as malaria, dengue, and Zika virus are amongst the largest healthcare concerns across the globe today. Each disease is transmitted by one or a few species of mosquito vectors and additionally, only females of the vector species can transmit the disease. To tackle such life-threatening diseases, it is vital to evaluate the risk of transmission. Of critical importance in this task is the estimation of vector species populations in an area of interest. Traditional approaches to estimating vector populations involve physically collecting vector samples in traps and manually classifying species, which is highly labor intensive. A promising alternative approach is to classify mosquito species based on the audio signal from their wingbeats.
Recently, a team of researchers from Faculty of Information and Communication Technology, Mahidol University (ICT Mahidol), explored the approaches to classify mosquito species and sex from their wingbeat signal. The team consists of Dr. Myat Su Yin, Prof. Dr. Peter Haddawy, Dr. Akara Supratak; three alumni students from ICT Bachelor program: Mr. Borvorntat Nirandmongkol, Mr. Tup Kongthaworn, and Miss Chanaporn Chaisumritchoke; a PhD candidate: Mr. Chaitawat Sa-ngamuang, and the Medical Entomologist: Asst. Prof. Dr. Patchara Sriwichai from the Faculty of Tropical Medicine.
In the research, “A Lightweight Deep Learning Approach to Mosquito Classification from Wingbeat Sounds”, what methods are used in classifying the species and genders of mosquitoes?
We employed a deep learning-based approach for mosquito species and sex classification from wingbeat sounds. Two lightweight deep learning models which are suitable to be deployed on small IoT sensor devices are implemented in our study. One model is a 1D CNN and the other combines the 1D CNN with an LSTM model. The models operate directly on a low-sample-rate raw audio signal and thus require no signal pre-processing. Both models achieve a classification accuracy of over 93% on a dataset of recordings of males and females of five species.
The methods we presented in our study are designed and tuned to address this specific problem of mosquito species and sex classification. Our approach might be applicable to the areas which have audio signals with similar characteristics with mosquito wingbeats audio such as bird classification or insect classification.
Those who are interested can see the full paper in ACM Digital Library: https://dl.acm.org/doi/10.1145/3462203.3475908.
Did you know? The research, “A Lightweight Deep Learning Approach to Mosquito Classification from Wingbeat Sounds” won the Best Paper Award from International Conference on Information Technology for Social Good (GoodIT 2021) which was held during September 9-11, 2021 in Rome, Italy.
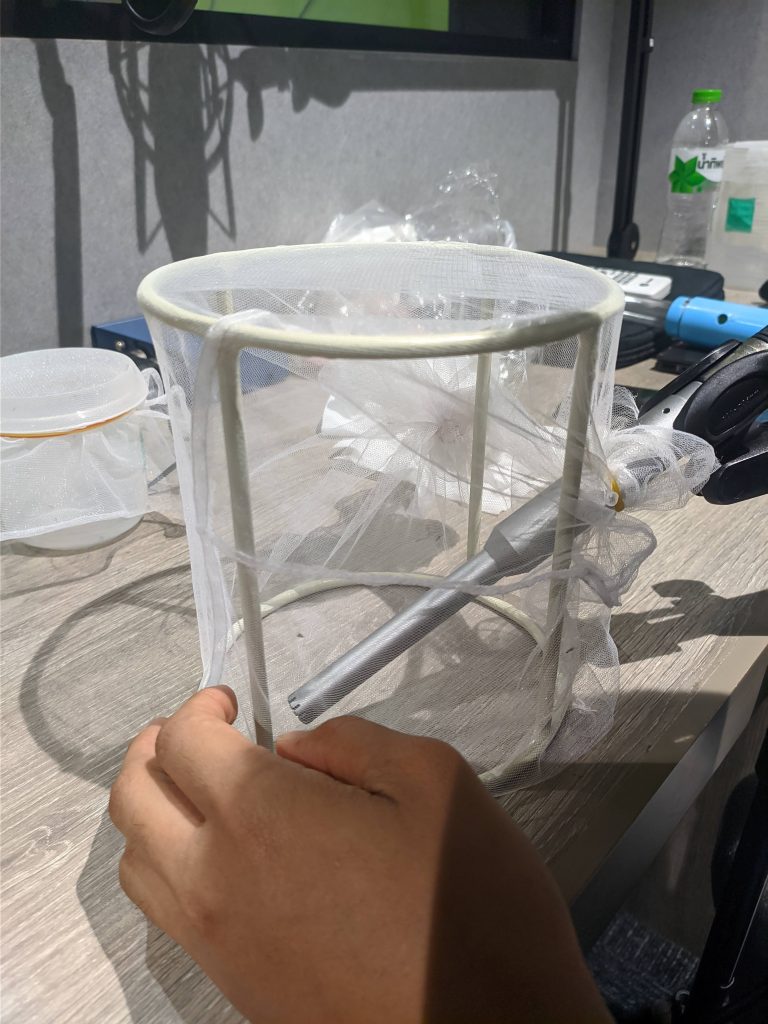